Need help with your Django project?
Check our django servicesNon-concurrent tasks in Celery & their potential caveats
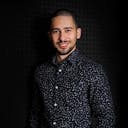
The problem
In HackSoft, we use Celery quite extensively.
Celery tasks can overlap in their execution (2 tasks, of the same type, running at the same time), but sometimes you don't want them - you want to prevent a task from being inserted into the queue, and executed, if an instance of that same task has is still running.
Meaning - sometimes we want to run only 1 task of a given instance, at a time, per queue. A non-concurrent task.
We have a mechanism for this in HackSoft, which we're sharing in the next sections of this blog post.
However, we recently caught an obscure bug that occurred due to our Celery configuration and it resulted in multiple running tasks, when there should be only one task in the queue at a given point in time.
Turns out, this is not an actual bug in Celery, but a specific configuration limitation which you need to be careful with when configuring such kind of infrastructure.
The non-concurrent tasks
By default, Celery does not have a built-in mechanism to say "This task should have only one running entry at a time in the same queue", meaning it is non-concurrent.
To handle this, we have implemented a custom task decorator:
import logging
from functools import wraps
from celery import shared_task
from myproject.tasks.apps import app as celery_app
inspect = celery_app.control.inspect
logger = logging.getLogger(__name__)
def non_concurrent_task(_func=None, *args, **kwargs):
def wrapper(func):
@wraps(func)
def inner(_bound_self, *_func_args, **_func_kwargs):
running_task_count = 0
queues = inspect().active()
if queues is None:
queues = {}
for running_tasks in queues.values():
for task in running_tasks:
if task["name"] == _bound_self.name:
running_task_count += 1
if running_task_count > 1:
logger.warning(f"[non_concurrent_task] Task {_bound_self.name} is already running")
return
return func(*_func_args, **_func_kwargs)
return shared_task(bind=True, *args, **kwargs)(inner)
if _func is None:
return wrapper
return wrapper(_func)
It ensures that if there's at least one task running in the queue - it won't dispatch a new one.
It iterates through the active queues, looks at the task names and if a task appears in a queue - a secondary dispatch is mitigated.
It is used in this way:
from myproject.tasks.decorators import non_concurrent_task
@non_concurrent_task
def custom_task(*args, **kwargs):
logger.info("A non-concurrent task is running")
import time
time.sleep(10)
logger.info("A non-concurrent task finished")
Then, spin up a Celery worker with the following command: celery -A myproject.tasks.apps worker
If you dispatch the non-concurrent task once, it will be processed by the worker as per usual. However, if you try to dispatch the same task a second time while the first execution is still not finished - you'll see a warning message from the decorator itself, saying that a task with such name is already running in this queue:
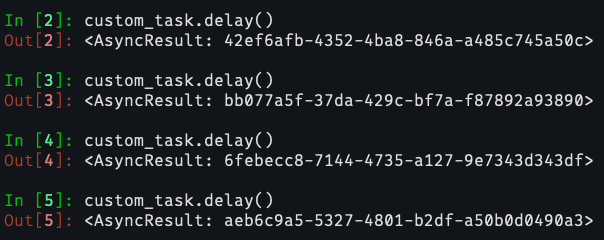

In the screenshots, you see that the first execution is started as expected, while the next 3 are "stopped" from the decorator, as the first one is not finished. The decorator does exactly what we want. Great.
Non-concurrent tasks and Celery worker names
Celery can distribute tasks across multiple worker processes, enhancing the application's scalability and preventing a single worker from getting overloaded.
As documented in Celery, you need to give each worker a unique name, using the -n
argument:
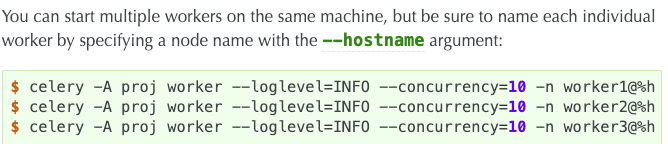
The easiest way to simulate this locally is by executing the command in multiple terminal sessions:
[Session 1] $ celery -A myproject.tasks.apps worker -n worker1@%h
[Session 2] $ celery -A myproject.tasks.apps worker -n worker2@%h
[Session 3] $ celery -A myproject.tasks.apps worker -n worker3@%h
This will span multiple Celery workers for the same queue. In this case, the default celery
queue.
If you want to specify the queue, just pass the-Q
argument to the command:celery -A myproject.tasks.apps -Q myQueue -n <workerName>
At this point, if you run celery inspect active
, you can see the currently active tasks. You can also get this programmatically using inspect().active()
:
{
'celery@worker1': [],
'celery@worker2': [],
'celery@worker3': [],
}
The easiest way to test this is by simulating a long-running operation in the dummy task with time.sleep(x)
. Or, if you have a real long-running operation that you want to be non-concurrent - use it directly in an existing task.
Then try executing the non-concurrent task more than once. It will still get "blocked" from the second execution onward, because the decorator does its job:
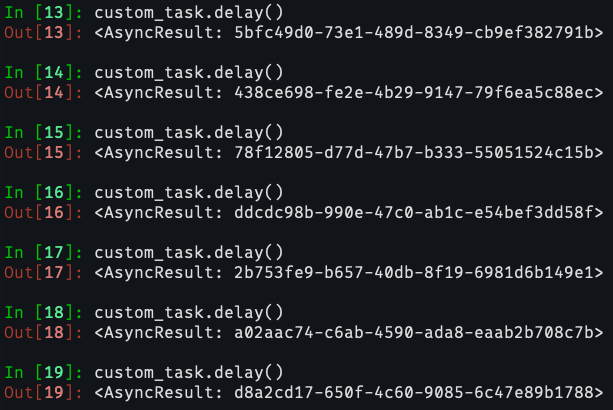
The very first task in the queue is obtained by the first worker.
All the next tries are being "stopped", even if they are processed by one of the other workers.



We were using this for quite some time and everything seemed fine. Up until the moment we started noticing one task being dispatched multiple times (thus overlapping with itself), even though the decorator seemed to be working.
At first, we started looking for the issue in the decorator, since it is a custom code that we've implemented. It turned out the issue is not within the decorator, but within our Celery configuration: we were using a non-unique identifier for the different workers.
So instead of:
[Session 1] $ celery -A myproject.tasks.apps worker -n worker1@%h
[Session 2] $ celery -A myproject.tasks.apps worker -n worker2@%h
[Session 3] $ celery -A myproject.tasks.apps worker -n worker3@%h
we were having:
[Session 1] $ celery -A myproject.tasks.apps worker -n worker
[Session 2] $ celery -A myproject.tasks.apps worker -n worker
[Session 3] $ celery -A myproject.tasks.apps worker -n worker
If you run the workers in this way, you will see the following warning (which is quite easy to miss, since Celery prints a lot of information when you initially start it):
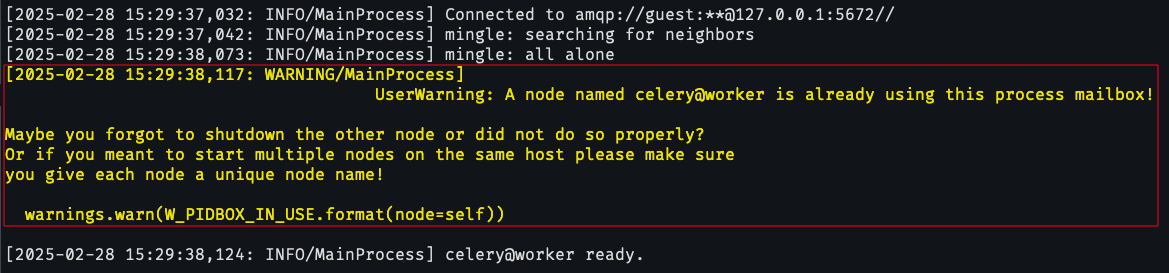
Here's the exact message:
Maybe you forgot to shutdown the other node or did not do so properly?
Or if you meant to start multiple nodes on the same host please make sure you give each node a unique node name!
However, since this is only a warning, the processes continue to run as normal.
This behaves quite strangely in Celery, though. If you run celery inspect active
in your shell, you will see the following:
{
'celery@worker': [...],
}
Since we use the same worker name for all processes, we see only one entry as active, while we expect to see three (the same number as the Celery processes we've dispatched).
Referring back to our @non_concurrent_task
utility decorator - we use inspect().active()
there to see what are the current active tasks in all queues and prevent a new one from being dispatched:
def non_concurrent_task(_func=None, *args, **kwargs):
def wrapper(func):
@wraps(func)
def inner(_bound_self, *_func_args, **_func_kwargs):
running_task_count = 0
queues = inspect().active()
if queues is None:
queues = {}
for running_tasks in queues.values():
for task in running_tasks:
if task["name"] == _bound_self.name:
running_task_count += 1
if running_task_count > 1:
logger.warning(f"[non_concurrent_task] Task {_bound_self.name} is already running")
return
return func(*_func_args, **_func_kwargs)
return shared_task(bind=True, *args, **kwargs)(inner)
However, when using explicit worker names and having them span multiple processes, Celery's inspect().active()
does not return idempotent results.
It does not differentiate between the processes that have the same worker name. A good experiment is to have a task (decorated with @non_concurrent_task
) and running two separate celery
processes for the given queue.
The result is that you can span multiple tasks in the same queue, concurrently, even though they're marked as non-concurrent:


You can clearly see that custom_task
is fully executed two times within a timeframe, while we expect it to be executed only once.
You may be guessing this already, but the solution in this case was quite simple - just use unique worker names when spanning multiple processes or omit the -n
flag completely if you can ensure unique hostnames in your environment (check the next section):
$ celery -A myproject.tasks.apps worker -n worker1@%h
$ celery -A myproject.tasks.apps worker -n worker2@%h
$ celery -A myproject.tasks.apps worker -n worker3@%h
Ultimately, this resolved the issue.
Rule of thumb: always take a second look at the documentation. :)
Caveats on Heroku
This specific app was deployed to Heroku.
Apps that are deployed to Heroku are configured via the so-called Procfile
. Simply said, it describes the processes you want to run when you deploy your app.
We have been using a Procfile
configuration like this for quite a while and everything was alright (or at least it seemed so):
worker: celery -A myproject.tasks.apps worker -Q celery
worker_2: celery -A myproject.tasks.apps worker -Q anotherQueue -n anotherWorker
worker_3: celery -A myproject.tasks.apps worker -Q yetAnotherQueue -n yetAnotherWorker
Each line in a Procfile
is a separate dyno (machine) in Heroku. Ultimately, the Procfile
shown above will result in three separate dynos.
Since the system handles a lot of tasks, we decided to span multiple dynos for each of these processes. Heroku made this as easy as a few clicks with the mouse.
But since we were using an explicit worker name without a unique identifier, the issue manifested itself.
However, since Celery is using the hostname as a default worker name if you don't give one explicitly.
This issue can be fixed on Heroku by completely removing the -n
flag from the configuration, because each dyno is a standalone machine, thus having a unique hostname.
Running celery inspect active
after that results in:
{
'celery@4b1729e4-392a-4e59-a104-deac929f7b8c': [],
'celery@5e82697e-48f9-43a1-b64f-b9c670eb39fd': [],
'celery@5000de24-e6e1-4567-a1f6-e8f127638705': [],
'celery@e69c4ea9-07e5-491e-bb0d-19da0b7a2631': [],
'celery@e4321513-9124-4fa7-b4da-defd9170fdd8': []
...
}
You can see that each hostname is a unique UUID, thus mitigating the issue.
Need help with your Django project?
Check our django services